Types of Uncertainty in Biostatistics
This post is about explaining the types of Uncertainty in Biostatistics, Aleatory, Epistemic and Ontological and why its important to quantify them in Clinical Research and Pharma Studies. Further, in this article i discuss these in more detail and some philosophical and practical aspects around the complexity of Uncertainty.
Darko Medin
7/5/20243 min read
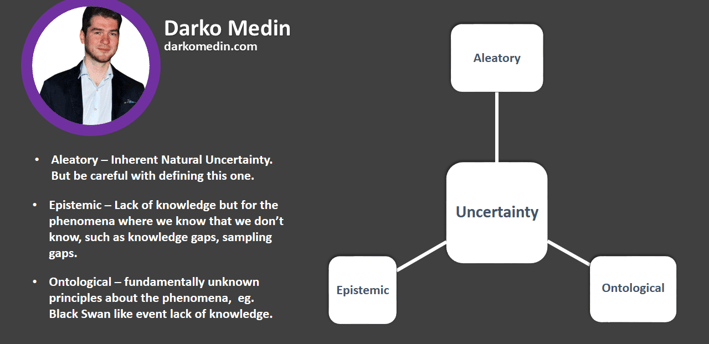
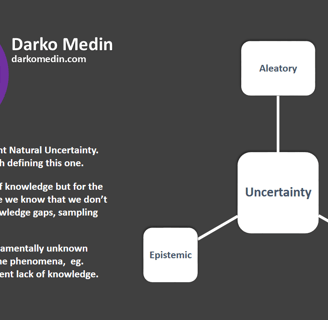
Uncertainty is one of the most important aspects to quantify in Biostatistics. It important for decision making in Trials, their design, interpretation but very important, its important from regulatory perspective. This is especially important in Life Science fields involving decision making such as Interim analyses in Clinical research, Analysis review by regulatory agencies, Platform trials, but also any other Clinical Study, because we need to assess Uncertainty in most fields.
The main definition exists for 3 types of Uncertainties, Aleatory, Epistemic and Ontological. But keep in mind that these are simplifications and the real world aspects are much more complex. In this article i will discuss some of those complexities.
Aleatory – This is considered as Inherent Natural Uncertainty, mostly related to Random / Stochastic processes and randomness in general. We know that most biological variables have their natural variability. For example number of T cells in immunology subjects will almost never be the same in the organism of those subjects. The PD-1 receptors per cell will also almost never be the same for a group of subjects and there is generally a range in which the values vary. On the other hand, instrument measurements may also have a level of randomness. But its important to distinguish the randomness and inaccuracy. A biochemical instrument may be accurate, but still have a level of variability if we were to make replicates of sample in a Biological study. If this variability is not attributed to inaccuracy and is small enough to be considered acceptable variability, it tends towards becoming the Aleatory, Inherent variability.
Epistemic – Lack of knowledge but for the phenomena where we know that we don’t know, such as knowledge gaps, sampling gaps. Sometimes we have a specific knowledge structure, and we know exactly where the knowedge gaps may be. In Clinical Trials its very important to understand potential knowedge gaps, such as those in sampling, variable presence, sample size and quantify uncertainty around them. This is the key in decision making in Clinical trials.
Ontological – fundamentally unknown principles about the phenomena, eg. Black Swan like event lack of knowledge. Black swan was an economical effect which was quite bad and no one really predicted it because most didn't predict that current most probable event, lack of fundamental knowledge which may be present. In Life science, this may be not having a significant part of the documentation for a measurement instrument or a biological process can be very dangerous to the project and is very important to avoid.
But be careful with defining uncertainty Scientifically and formally. If we take the approach of 'Laplace's super-being' (i called it superbeing to be more subtle than Laplace, although he used a different term), thinking about Uncertainty gets more interesting. According to the famous mathematician, Laplace thought experiment, if a supe-being that knew everything about everything in universe existed, it could predict everything [Laplace 1902, A Philosophical Essay on Probabilities] to the level of being able to predict anything, atoms movement, Brownian motion, anything even a role of a dice, my example : it would be able to do this based on knowing the exact 3d positioning of the dice, its kinetic energy received by fingers and the all other additional information such as air resistance and so on. So, it would be able to predict a dice roll, which is for a real world unpredictable, a part of the random variable. So if all information was there, it would be predictable, but due to practically being unpredictable, it is considered as random, but for the reason of having no practical way to add predictive information about the phenomena.
Formally, my opinion all uncertainty is lack of information and Laplace is correct formally, but practically, we can indeed separate them in Aleatory, Epistemic and Ontological and pay attention to in Clinical and Biological Research, as we frequently just dont have all the information and the reality is quite complex there.